Four hundred years.
That’s how long humanity has been attempting to mathematize fairness. The seeds for the idea of algorithmic fairness were sown during the Enlightenment as part of society’s shift from religiously grounded ethics to decisioning frameworks based on reason. This transition fostered the belief that questions of fairness could be objectively determined and mathematically modeled.
One of the earliest examples can be found in the 17th century, with the development of the concept of “present value.” Mathematicians grappled with the question of how to determine the current worth of a future asset, a problem that arose in the 1620s during negotiations over the potential union of England and Scotland. The question of how much Scottish landowners should be compensated by the English government for future tax increases demanded a calculation of present value.
This early example illustrates a recurring theme: the use of mathematical tools to resolve questions of fairness. The development of probability theory, for instance, was driven in part by the desire to fairly divide winnings in gambling and to assess the risks and rewards associated with uncertain ventures like insurance and shipping. The question of what constituted a fair distribution of risk and reward in these early financial instruments set the stage for all our modern debates about fairness in lending, insurance, and other algorithmic decision-making processes.
To be sure, throughout this long history, debates have raged over the definition of algorithmic fairness. Some argue for redistributive definitions of fairness, where those deemed “low risk” help offset the liabilities of those considered “high risk.” Others advocate for anti-redistributive definitions of fairness, prioritizing individual responsibility and minimizing the pooling of risk. These competing conceptions of fairness continue to shape contemporary discussions about algorithmic bias and discrimination.
But the pursuit of algorithmic fairness is not a new phenomenon. It is an aspiration that has reappeared again and again throughout history, as thinkers apply mathematical reasoning to resolve complex social and economic problems. Understanding this long history will be critical to navigating the difficult debates our society will face over algorithmic fairness in the years to come.
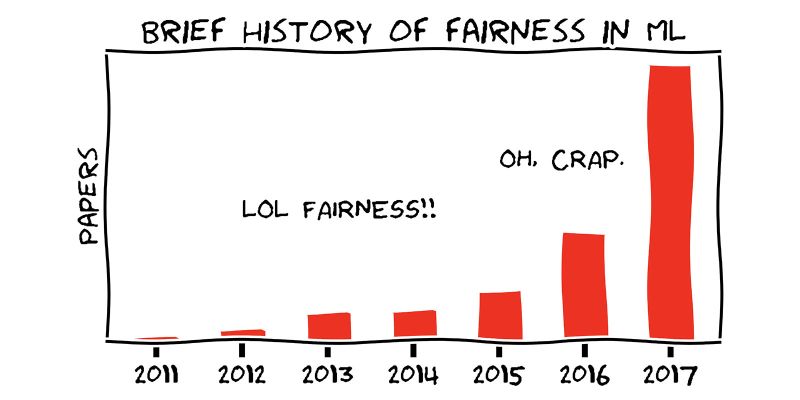